Data is at the core of modern enterprises. Organizations produce vast amounts of information daily but often struggle to harness its power effectively. That’s where Deploying data fabric comes into play. This innovative architecture is designed to streamline data access and management across different environments. Yet, deployment is not without challenges. This post explores some common obstacles faced by data engineers and enterprise solution architects when trying to implement one, providing actionable strategies to overcome any hurdles they encounter along the way.
Understanding Data Fabric
Data fabric is an architectural framework that offers a centralized solution for data management across various environments (on-premises, cloud, or hybrid), allowing seamless integration, access management, and analysis for better decision-making and analytics in large organizations where disparate sources often obstruct comprehensive analysis.
Data Modeling Is Essential
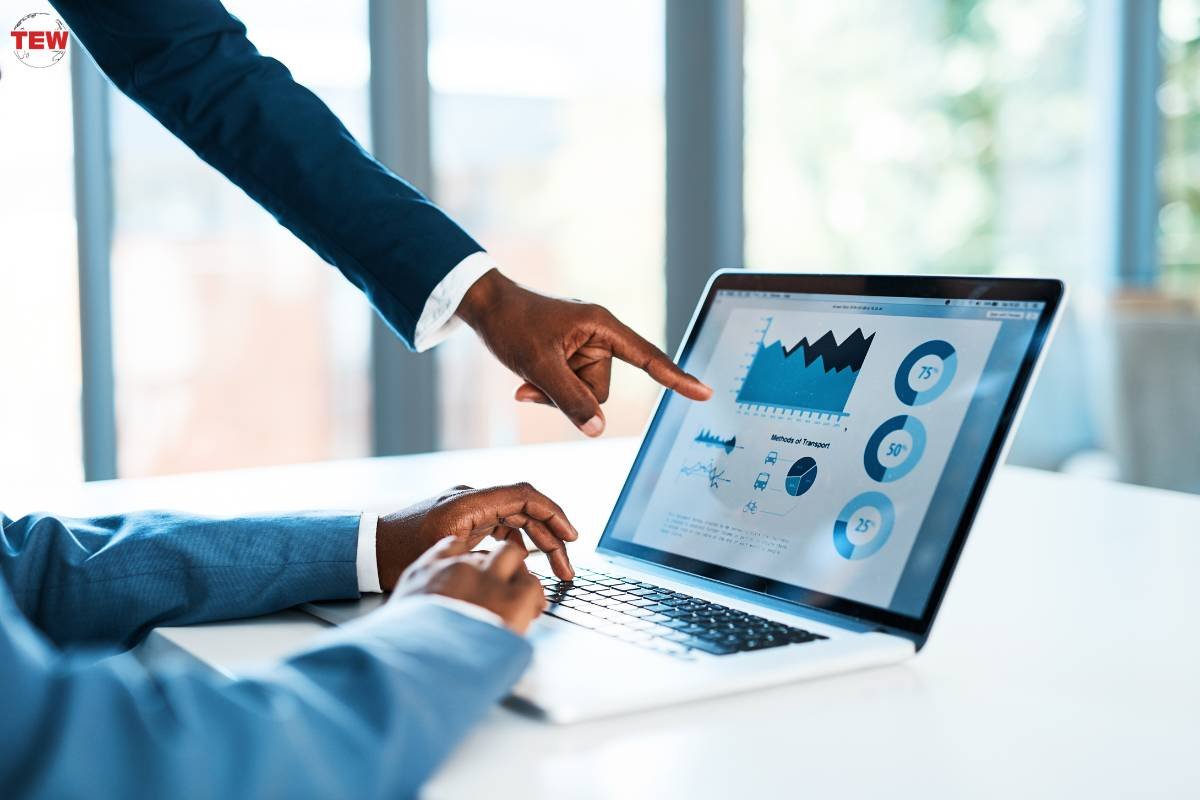
One fundamental component of data fabric implementation is data modeling. An effective data model sets the stage for how data will be organized and accessed. This can be especially important for large enterprises as it determines its structure and flow within an organization. This leads to enhanced insight and efficiency gains.
However, creating a data model tailored to meet the needs of a large organization is no simple task. Data engineers often struggle with various data formats, structures, and standards across departments. Dedicating time and resources toward creating an inclusive data model can reduce these headaches and enable smoother fabric deployment.
Common Challenges in Deploying Data Fabric
Deploying data fabric can present many obstacles that impede its successful implementation, from technical difficulties to compliance issues. This section will explore some of the most frequently faced challenges during data fabric deployment and offer insight into how organizations can address them effectively. By understanding these difficulties better, enterprises can prepare themselves for what lies ahead while developing an ideal data fabric solution to suit their unique needs and objectives.
1. Data Fabric vs. Data Mesh
To understand modern data solutions, it is vital to differentiate between two prevalent concepts: data fabric vs data mesh. Both approaches seek to address the complexity of managing vast amounts of information across organizations but take different approaches in doing so.
Data Fabric focuses on creating an integrated and unified architecture that enables seamless connectivity and access to different data assets, as well as their orchestration by automation, metadata management, and intelligent data orchestration to provide an overall view of an organization’s data landscape. Data fabric typically relies on overarching policies as its guidepost for successful operation across diverse environments. For instance, like scaling capacity and interoperability across diverse data environments.
Data Mesh advocates for a decentralized and domain-centric approach. It decentralizes data ownership by delegating its management to individual teams or domains, with each managing its data independently to foster greater accountability and agility. Data mesh employs principles such as domain-driven design, product thinking, and federated governance to ensure data management is closer to its source, thus improving responsiveness and scalability.
Data fabric emphasizes a centralized data architecture, while data mesh favors decentralized, domain-centric approaches. Organizations must carefully consider their individual requirements, culture, and operational objectives before selecting an approach best aligned with their data management and analytics goals.
2. Data Silos
A key challenge of successful data fabric deployment is data silos. Different departments often operate independently within an enterprise, creating isolated datasets that are difficult to integrate. Such silos create poor accessibility of information as well as redundant efforts and conflicting interpretations.
3. Legacy Systems
Legacy systems present another significant obstacle in deploying data fabric. Many large enterprises rely on obsolete technologies that cannot meet modern data integration demands, which may slow data processing speeds, increase maintenance costs, and create compatibility issues between legacy and newer technologies.
4. Data Quality Issues
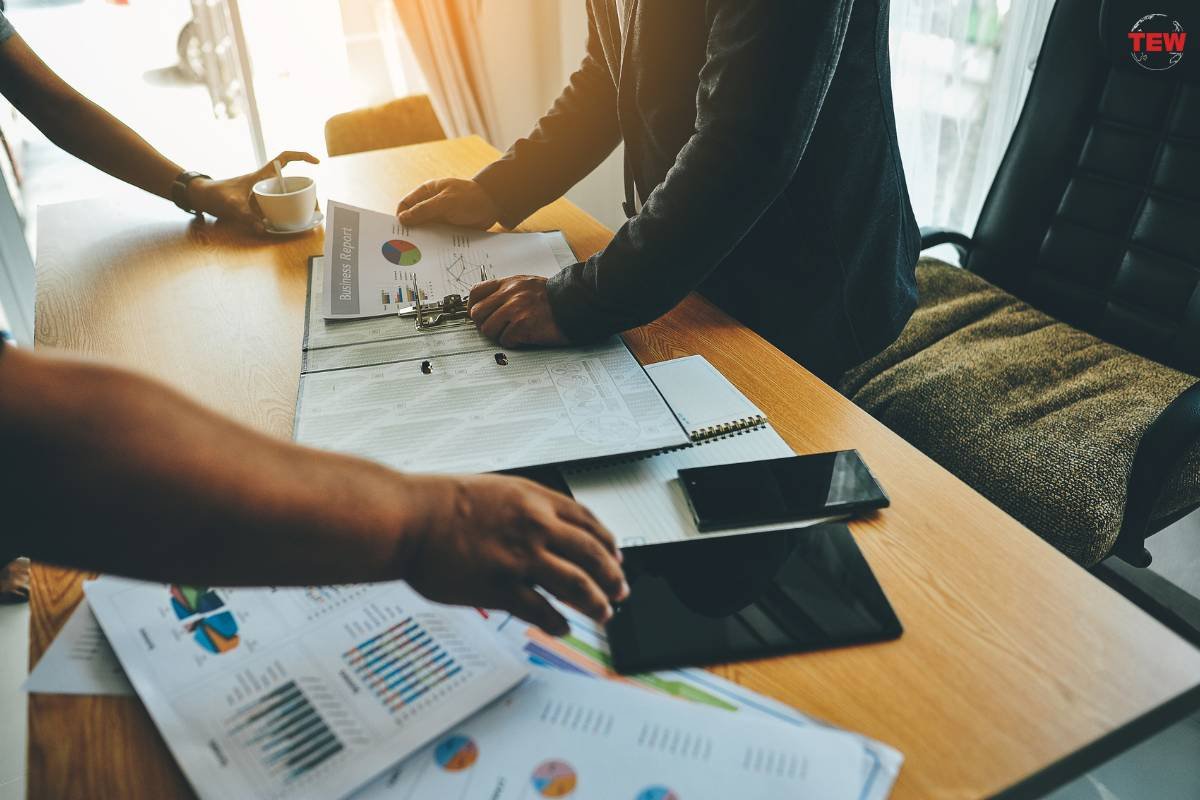
Achieve success when implementing a deploying data fabric implementation requires quality data. Poor-quality information can lead to inaccurate analyses and lead to misguided business decisions. Large organizations often face difficulty maintaining uniform data quality across diverse datasets due to differing entry, storage, and processing methods.
5. Solutions to Overcome Deployment Challenges
Implementing a data fabric strategy may present numerous obstacles, but with the appropriate solutions these obstacles can be overcome successfully. Anticipating potential issues early will allow organizations to streamline their integration processes while making data easily accessible, reliable, and actionable. This section will examine practical strategies for overcoming some of the more commonly experienced deployment difficulties of data fabric, such as managing legacy systems, assuring data quality, or optimizing technology stack.
6. Implementing a Unified Data Management Strategy
Unifying data management strategies is essential to overcoming deployment obstacles. Organizations should strive to develop an overarching framework that balances governance, quality, and accessibility of their data assets. A good data management strategy also involves clearly delineated roles and responsibilities as well as protocols for integration and sharing of the collected information.
Adopting a centralized data management platform is one effective strategy, as this enables data engineers and solution architects to monitor and control data flows from one central interface, making it simpler to manage diverse datasets. Furthermore, centralizing data management facilitates real-time collaboration among teams, thereby breaking down silos and cultivating a data-centric culture.
7. Utilize Advanced Technologies
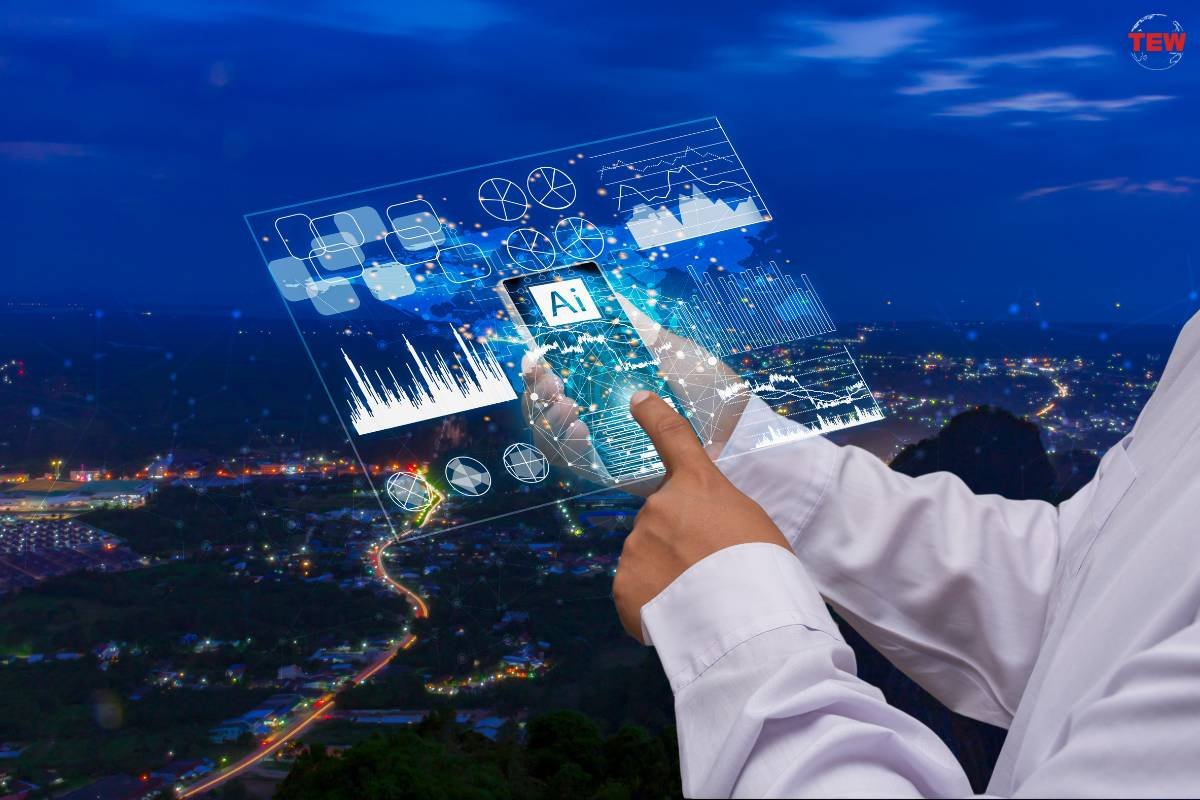
Implementing advanced technologies, like AI and machine learning, can enhance the success of data fabric deployment. Such technologies help automate data integration, quality checks, and anomaly detection while relieving engineers of their duties and increasing overall efficiency.
Cloud-based solutions can significantly enhance scalability and flexibility for large enterprises, giving them easier access to vast datasets stored and processed on the cloud. Leveraging modern technology ensures their data fabric remains robust and future-proof.
8. Continuous Monitoring and Improvement
Implementing data fabric requires constant oversight and refinement. Organizations should establish key performance indicators (KPIs) to gauge its success. Regular assessments of data quality, accessibility, and user satisfaction can help pinpoint areas for improvement.
Establishing a culture of continuous learning within an organization can significantly boost the deployment process. Hosting training sessions and workshops on emerging data trends, technologies, and best practices enables data engineers and solution architects to continually optimize their approach.
Conclusion
Implementing data fabric can be an arduous yet transformative journey that reshapes how organizations manage, integrate, and use their data. By centralizing data management and taking advantage of advanced technologies while making commitments towards continuous improvement, businesses can achieve an agile data-centric environment characterized by reduced silos, enhanced quality data, and greater scalability.